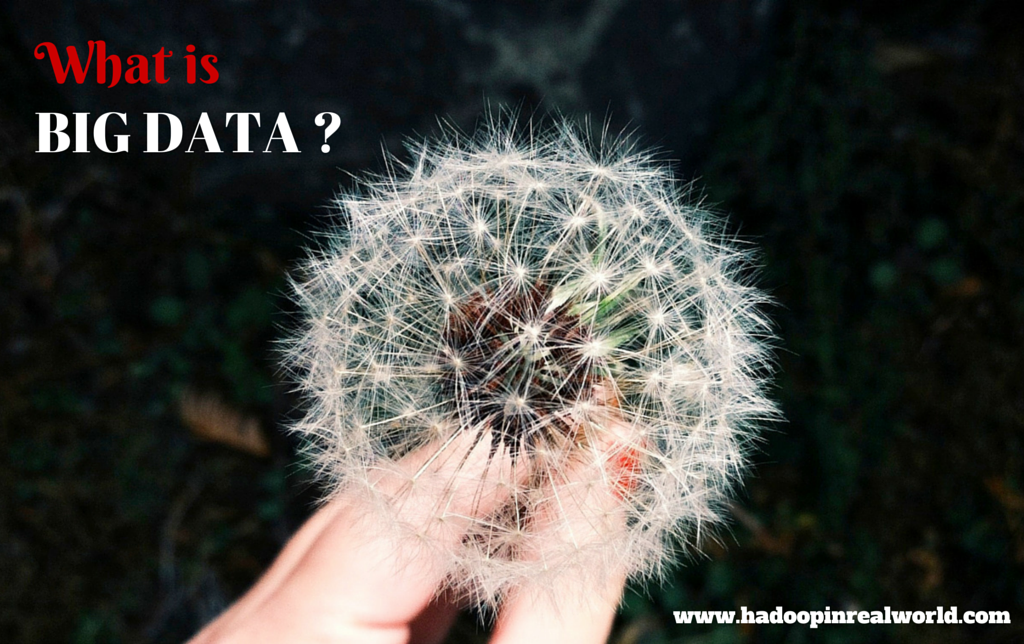
What is Big Data?
June 19, 2015What is DistCp?
June 25, 2015What is Hadoop? – A beginner’s tutorial to understand Big Data problem and Hadoop
In this Post we looked at What is Big Data. To learn about what is Hadoop? we need to first understand the challenges with big datasets in terms for storage and computation. Once we understand the problems we are trying to solve we can easily understand the solution – which is Hadoop.
Let’s take a sample big data problem, analyze it and see how we can arrive at a solution together. Ready?
Imagine you work at one of the major exchanges like New York Stock Exchange or Nasdaq. On morning someone from your Risk department stops by your desk and asked you to calculate the maximum closing price of every stock symbol that is ever traded in the exchange since inception. Also assume the size of the dataset you were given is 1 TB.
Immediately the business user who gave this problem asks you to give an ETA on when he can expect the results.
Wow!! There is lot of think here to answer that question. So you ask him to give you some time and you start to work. What would be your next steps?
We need to figure out 2 things – The first one is how to store this dataset and the 2nd how to perform the computation. Lets talk about storage first.
Your workstation has only 20 GB of free space, so you go to your storage team and ask them to copy the dataset to a Network Attached Storage or NAS or SAN server and ask them give you the location of the dataset. A NAS is a data storage server connected to your network so any computer with access to the network and the NAS server can access the data if they are permissioned to see the data. So far good. The data is stored and you have access to the data and now you set out to solve the next problem which is computation.
You are a Java programmer so wrote an optimized Java program to parse the file and perform the computation. Everything looks good and you are ready execute the program against your dataset.
You realize it is already noon, the business user who gave you this request stop by for an ETA. That’s an interesting question, isn’t? For the program to work on the dataset, first the dataset needs to be copied from the storage to the working memory or RAM. So estimate the execution time you need to know the time to read the dataset from the hardisk and how long will it take to compute the result.
So how long does it does to take to copy a 1 TB file from storage ?
Let’s take our traditional Hard Disk Drives (HDD), that is the one that is attached to our laptop or workstation. HDDs have magnetic platters in which the data is stored. When you request to read data, the head in the hard disk first position itself on the platter and start transferring data from the platter to the head. The speed in which the data is transferred from the platter to the head is called the data access rate.
Average data access rates in HDDs is usually about 122 MB per secs. if you do the math, to read 1 TB file from a HDD you would need about 2 hr and 22 mins.
Now that is for a HDD that is connected to your work station. When you transfer a file from the NAS server you should know the transfer rate of the hard disk drives in the NAS server. For now we will assume it is same as the a regular HDD which is 122 MB and hence it would take 2 hrs and 22 mins just to read the data from the hard disk. While you were calculating all this the business user is still standing waiting for a ETA 🙁
Now, what about computation time? Since you have not executed the program yet atleast once you can not say that for sure plus your data comes from a storage in the network so you have to consider the network bandwidth also. With all that in mind you tell him the ETA is about 3 hours but it could be easily over 3 hours since you are not sure about the computation time.
Your user is shocked to hear 3 hours and comes next question. “Can we get it sooner than 3 hrs say may be in 30 mins?” You know there is no way you can get the result in 30 mins. Of course business can not wait for 3 hours, especially in finance where time is money. 3 hours is unacceptable.
So lets work this problem together. How can we calculate the result in less than 30 mins or less?
Lets break this down. Majority of the time you spend in calculating the result set will be attributed to 2 tasks.
- Transferring the data from the storage or Hard Disk drive which is about 2 and half hours and
- Computation time – that is the time to perform the actual average close price by your program. Let’s say it would take 60 minutes.
Crazy idea! To reduce the time to read the data from hard disk, what if we replace HDD by SSD? Solid State Drives or SSDs are very powerful alternatives to HDD. SSD does not have magnetic platters or heads and they don’t have any moving components and they are based on flash memory and hence extremely fast.
Sounds great. Why don’t we use SSD in place of HDD?
SSD comes with a price. They are usually 5 to 6 times in price than your regular HDD. Although the prices continue to go down over time given the data volume that we are talking about with respect to big data, it is not a viable option now. So for now we are stuck with HDDs. Let’s talk about how we can reduce the computation time. Hypothetically we think the program will take 60 mins to complete and let’s also assume the program is already optimized for execution.
What can be done next? Any ideas? I have a crazy idea!!
How about dividing the 1 TB dataset in to 100 equal size chunks or blocks and have 100 computers or nodes do the computation parallely?
In theory, this means you cut the data access rate or speed with which you read by a factor of 100 and also the computation time by a factor of 100. So with this idea, you can bring the data access time to less than 2 mins and computation time to .6 mins. Beautiful!!
It is a promising idea so lets explore even further.
There are couple of issues with this idea. If you have more than one chunk of your dataset stored in the same hard drive you can not get a true parallel read because you have only one head in your hard drive to read the data. But for the sake of argument, let’s assume you get a true parallel read, which means you have 100 computers reading the data at the same time. Now assuming the data can be read parallely you will now have 100 times 122 MB / sec of data trying to flow through the network.
Imagine this, what would happen when each one of your family member at home starts to stream their favorite TV Show or Movie at the same time using the single internet connection you have at your home?
It would result in a very poor streaming experience with lot of buffering and no one in the family can enjoy their show. What you have essentially done is choked up your network. The download speed requested by each one of the devices combinely exceeded the download bandwidth offered by the internet connection resulting in a poor service. That is exactly what will happen here when 100 nodes tries to transfer the data over the network at the same time.
How can we solve this?
Why do we have to rely on a Network Attached Storage? Why don’t we bring the data closer to computation. That is, let’s store the data locally in its own hard disk in each node. So you would store block 1 of data in node 1, block 2 of data in node 2 etc..
Now we can achieved a true parallel read on all 100 nodes and also we have eliminated the network bandwidth issue.
Now that we have divided our data in to blocks, our exposure to data loss increases because when one of the 100 nodes goes down we would lose the data stored in our node. We would also require all 100 chunks or blocks of data to calculate the maximum closing price. Losing a block of data will result in incomplete result. In reality, data loss is unavoidable even in a single node situation but we need a way to recover from the data loss.
Data Loss
I am sure most of us at least once were in a hard drive failure situation and it is no so much fun. So how can you protect your data from hard disk failure, data corruption etc. ?
Let’s take an example. Let’s say you have a photo of your loved ones and you treasure that picture. In your mind there is no way you could lose that picture. How Would you protect it? You would keep copies of the photo in different places right – may be one in your laptop, one copy in picasa, one copy in your external hard drive. you get the idea.. So if your laptop crashes you can still get that picutre from picasa or your external hard drive.
We can do something similar for our data loss situation. Lets do this, why don’t we copy each block of data to 2 more nodes or in other words we can replicate the block in 2 more nodes. So in total we will have 3 copies of the block.
Take a look at below. Node 1 has block 1, 7 and 10. Node 2 has blocks 7, 11 & 42. Node 3 has blocks 1, 7 and 10. So if block 1 is unavailable in node 1 due to a hard disk failure or corruption in the block, it can be easily fetched from node 3.
So this means that node 1, 2 and node 3 must have access to one another and they should be connected in a network, thus forming a 100 node cluster. Conceptually this is great but it has some challenges implementing it.
How does Node 1 knows that Node 3 also has Block 1? Who decides Block 7 should be stored in Node 1, 2 and 3? First of all who will break the 1 TB in to 100 blocks? This solution does not look easy isn’t? and that is just the storage part of it.
Computation brings other challenges. Node 1 can only compute the maximum close price from just block 1, node 2 can only compute the maximum close price from block 2. This brings up a problem because for eg.. data for stock GE can be in block 1 and also in block 2 and could also be on block # 82. So you have to consolidate the result from all nodes together to computer the final result. Who is going to coordinate all that?
The solution we are proposing is distributed computing and as we are seeing it has several complexities to implement both at the storage layer and also at the computation layer.
The answer to all those open questions and complexities is Hadoop. Hadoop offers us a frame work for distributed computing. Hadoop has 2 core components – HDFS & MapReduce.
HDFS
HDFS stands for Hadoop Distributed File System and it takes care of all your storage related complexities like splitting your dataset into blocks, takes care of replicating each block to more than one node and also keep track of which block is stored on which node.. etc.
MapReduce
MapReduce is a programming model and it takes care of all the computational complexeties.. like bringing all the intermediate results from every single node to offer a consolidated output…
What is Hadoop?
Hadoop is framework for distributed processing of large datasets across clusters of commodity computers. The last 2 words in the definition is what makes Hadoop even more special. “Commodity computers” means that all the 100 nodes in the cluster that we talked about does not have any specialized hardware. They are enterprise grade server nodes with a processor, hard disk and RAM in each of them but other than that nothing special about them. Don’t confuse commodity computers with with cheap hardware it means inexpensive hardware but not cheap hardware.
Now you know what Hadoop is and how it can offer an efficient solution to your Maximum close price problem against the 1 TB dataset. Now you can go back to that business user and propose Hadoop to solve the problem and to achieve the execution time that your users are expecting. But if you propose a 100 node cluster to your business expect to get some crazy looks. But that is the beauty of Hadoop, you don’t need to have a 100 node cluster. We have seen Hadoop production environments from small 10 nodes clusters all the way to 800 to 1000 nodes clusters. You can simply start even with a 10 node cluster and if you want to reduce the execution time further all you have to do is add more nodes to your cluster. That simple. In other words Hadoop will help you horizontally scale.
Now you know what is Hadoop and conceptually how it solves the problem of big datasets.
1 Comment
[…] View Image More Like This […]